Single-cell sociobiology
Alex K. Shalek's lab seeks to understand function and dysfunction within cellular communities
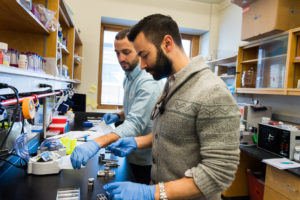
Alex Shalek (right) and graduate student Marc Wadsworth (left) prepare samples for future single-cell studies using the Seq-Well technology. Credit: Justin Knight
Experimental tools and computational advances enable us to observe individual cells like never before. But, if we are made of trillions of cells, why does getting to know any single cell matter?
The health of our tissues — such as skin, intestine, liver, or lung — depends on the combined and coordinated behaviors of vibrant cellular communities. For example, many specialized cell types must effectively collaborate with one another to protect against a virus attempting to gain residence in our lungs. Certain lung cells, whose primary jobs are to mediate efficient oxygen exchange, now have to decide if they are able to thwart a viral invasion themselves, or if they need immune cell reinforcements. Invoking the cavalry, in turn, requires the right set of checks and balances to ensure that the response is of sufficient vigor (to avoid immunodeficiency) and that only the diseased cells are targeted for destruction, especially if it’s a false alarm (to avoid autoimmunity). Identifying the implicated cell types and the molecular signals that help ensure appropriate responses is paramount to improving diagnostics, therapeutics, and prophylactics for a wide variety of diseases.
Until recently, to reveal the properties and unique roles of a tissue’s component cells, scientists binned them into subgroups from the “top down,” based on known features, and then characterized each subgroup en masse. Improvements in measurement technologies, such as the advent of genome-wide profiling methods like RNA sequencing, now allow us to collect information on everything a subgroup of cells intends to do at a given moment. Yet, as powerful as these approaches have become, they fundamentally do not allow us to see whether each individual cell that fits a prospective definition is actually behaving the same way.
We needed new tools that would allow us to understand both the common and unique attributes of each cell to avoid generalizations and a groupthink mentality. Building a framework directly from observations of individual cells — a “bottom-up” approach — clarifies the true diversity among cells and the usefulness of different classifiers.
My team seeks to understand the overarching principles that inform how cells and their interactions drive systems-level behaviors.
A “bottom-up” revolution
Within the last decade, converging advances in molecular biology, nanotechnology, optics, electronics, and computer science have made it possible to apply RNA sequencing to individual cells. By examining everything a cell is thinking and then computationally identifying how distinct thoughts are linked across cells, we helped prove that we could find cellular thought patterns that revealed valuable groupings and uncover attributes associated with them, such as common motivators.
Rather than testing hypotheses individually, we could now learn directly from the cells about the patterns that best described their actions in complex tissue communities. This advance promised a data-driven revolution in basic and applied biology. Just imagine how comparing healthy and diseased tissues in this way would let us discover the relevant cellular and molecular differences that enable cancer cells to survive treatment or that contribute to an autoimmune condition.
However, initial studies took weeks to months and hundreds of dollars per cell. Realizing the potential of these “bottom-up” approaches required high-throughput methodologies that could sample the cells that form these complex tissue communities, as well as achieve the statistical power necessary to overcome the noise inherent in measuring minute quantities of RNA.
In collaboration with labs at Harvard University, Harvard Medical School, and the Broad Institute, we devised Drop-Seq, an approach that barcodes cells prior to performing any other processing, allowing us to pool together thousands of cells for subsequent manipulation and profiling while still enabling us to recover each measurement’s cellular source. Now, we could profile thousands of single cells and identify their attributes directly from the data at costs of cents per cell in one day. However, the Drop-Seq suffered from cost, portability, sample input, and scalability limitations that hindered the types of questions we could ask and where we could ask them.
In 2017, in partnership with J. Christopher Love’s lab at MIT, we overcame these issues with Seq-Well, a redesign that replaced the microfluidic chip and complex equipment used to barcode cells in Drop-Seq with an array of picoliter-sized wells, a membrane, and a clamp. This simplification allows us to process tens of thousands of cells a day anywhere in the world. For example, with collaborators from the Philip T. and Susan M. Ragon Institute, we have traveled to South Africa to analyze tissue and blood samples to determine which cells can restrict the outgrowth of tuberculosis or help control HIV-1 virus.
Translating single-cell RNA sequencing to the clinic
Most recently, with collaborators at Brigham and Women’s Hospital, we applied Seq-Well to profile and molecularly define more than 50,000 cells that lined the nasal cavity in the hopes of identifying new treatments for a severe form of allergic inflammation called chronic rhinosinusitis (CRS). CRS affects 12 percent of Americans and often results in recurring polyps in the sinus cavity that can lead to painful sinus pressure, difficulty breathing, and a loss of smell. While it has long been appreciated by peering through a microscope that these polyps are characterized by accumulations of basal stem cells, two fundamental questions remained unanswered: How are these stem cells distinct from those that can differentiate to form healthy nasal tissue?; and how might they contribute to the chronicity of the disease?
We found that basal cells from polyp tissue contain changes not seen in their non-polyp counterparts that may effectively lock them into a stuck state: neither a stem cell nor a productive differentiated cell type. The common driver for these changes related to specific cytokines (instigating inflammatory molecules generated by immune cells), and persisted for weeks in surgically removed basal cells in the lab. These basal stem cells replenish themselves and differentiate to generate several key cell types necessary to form healthy nasal tissue, so their memories can be passed down to both their stem and differentiated progeny, resulting in near-permanent changes to the tissue they replenish. This unexpected finding would have been missed via topdown analyses, and reveals basal stem cells as key cells-of-origin for the persistence and chronicity of human allergic inflammation.
Our finding suggested that to treat this inflammatory disorder, we needed to block improper immune responses and work to remove any remaining memory of previous insult. Since Seq-Well is compatible with low-input samples, we were able to examine the effects of a therapeutic antibody targeting the relevant immune responses in a CRS patient by scraping their nasal polyps before and after treatment. After a month and a half of therapy, we found a substantial restoration of gene expression associated with healthy tissue, confirming our hypothesis. Some differences remained, however, which suggested new candidate targets for future therapies.
Sequel to Seq-Well
Overall, our study may provide an explanation for why other chronic inflammatory disorders are often so intractable and difficult to treat, and point to new therapeutic avenues. When our immune systems mis-police our tissues, they compensate in unexpected ways. Restoring order to our tissue’s immune cells helps, but it doesn’t necessarily guide non-immune cells to forget the past.
Could a history of environmental insult drive catastrophic responses, such as cancer, and suggest new classes of early interventions? We don’t know yet, but at least we now know how to look. Early work in adult ulcerative colitis with labs at Massachusetts General Hospital and the Broad Institute initiated through the Center for Microbiome Informatics and Therapeutics at MIT suggests the answer may be yes. In addition, studies of pathogen-induced changes to gut tissue in Zambia and South Africa with labs at Barts and the London School of Medicine and Dentistry and the Africa Health Research Institute also provide important pieces of the puzzle. To more thoroughly follow leads, we’re currently developing improved model systems that will allow us to perform rapid screens for testing and prioritizing new treatments.
Fundamentally, my team seeks to understand the overarching principles that inform how cells and their interactions drive systems-level behaviors. We believe that achieving a working knowledge requires experimental and computational strategies that can examine and control individual cells, their microenvironments, and their interactions, allowing us to isolate the contributions of each. Appreciating the complexities of different cellular systems, developing the correct experimental and computational tools to study them, and properly applying those approaches requires, in turn, an interdisciplinary team composed of the best and brightest across MIT’s technical strengths that can, itself, do more than the sum of its parts. I consider myself fortunate to have the opportunity to draw from the breadth and depth at MIT — graduate and undergraduate — to nucleate a unique environment that allows us to pursue projects that would be difficult to conceive elsewhere.