Researchers Explore Mutual Benefits of AI and Science
MIT scientists are leveraging advances in computing to answer increasingly complex questions while contributing to the next generation of artificial intelligence tools.

ChatGPT’s launch in 2022 brought artificial intelligence into the mainstream. But the vast and rapidly evolving set of tools encompassed under the AI umbrella began transforming health care, transportation, and more, long before that — thanks, in part, to the efforts of MIT researchers.
Across the Institute, researchers are using AI to build advanced robots, predict group decisions, and identify anomalies in unwieldy datasets. Biologists are using AI to annotate medical scans. Chemists are using it to interpret the structure and function of molecules. Cognitive scientists are using large language models, a type of AI trained on text, to understand the basis of human language. And that’s just the beginning.
But the integration of science and AI is a two-way process. Scientists are utilizing these tools to digest large datasets and ask increasingly complex questions, but they’re also leveraging fundamental scientific concepts to build more interpretable and efficient AI for a range of applications.
“If one can express scientific problems in the abstract language of computation, one can blur the boundaries between disciplines and leverage AI advances to tackle problems across both AI and science.”
The National Academies described it as the “symbiotic relationship” between research and AI in a recent neuroscience workshop report.
That symbiotic relationship is driving questions around behavior, vision, language development, and more as part of the MIT Quest for Intelligence, directed by James DiCarlo, Peter de Florez Professor of Neuroscience.
“The unique thing we are doing is that the engineering systems that we are developing are aiming to be both the next generation of AI models and the next generation of scientific models of brain function,” DiCarlo says.
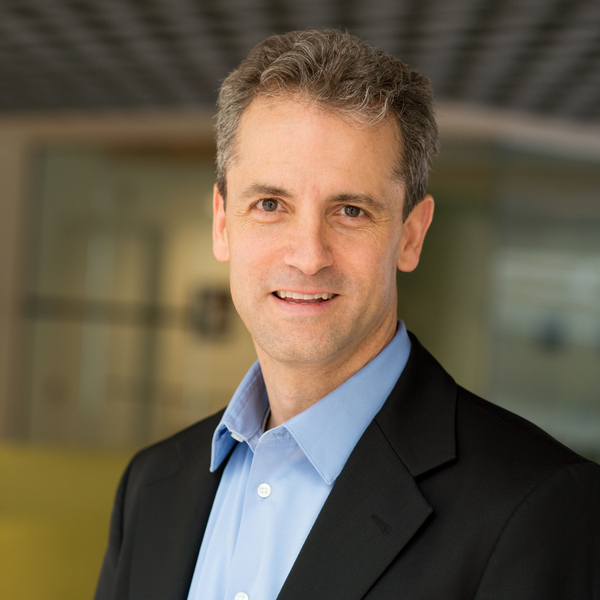
Machine learning, a subset of AI where algorithms learn — even in the absence of encoded instructions — was largely inspired by the brain’s neural networks and is useful for processing data and for exploratory research on the basis of natural intelligence.
DiCarlo’s lab, for example, uses machine learning to understand how humans create and interpret visual images. They’re building artificial networks that replicate the brain’s architecture to study the mechanisms behind visual representation while applying that research to produce AI tools that can effectively perform visual tasks. One day, those discoveries could inform the development of brain-machine interfaces to restore lost vision.
“Before recent AI methods and tools were available, we didn’t even have approximate computational models of brain sensory systems,” DiCarlo says. “Modern machine learning methods are now producing the leading scientific models of brain function.”
AI methods are also being applied in fields that have long relied on computational methods. Noelle Selin, professor in the Institute for Data, Systems, and Society and the Department of Earth, Atmospheric and Planetary Sciences, models trends in air pollution and climate change to inform decision-making. AI is enabling scientists like Selin to make more accurate projections and better account for climate variability and societal interactions in those models.

The new Center for Sustainability Science and Strategy, directed by Selin, also aims to incorporate AI into existing MIT-based tools like its Integrated Global Systems Modeling framework, which provides information on combined risks from social and environmental hazards.
“The real promise and impact of AI tools and methods is just beginning to be applied in climate modeling and sustainability science,” Selin says.
In addition, AI could provide a valuable “productivity boost,” Selin says, by making it easier to process the vast amounts of data — tens of millions of iPhones worth — output by climate models.
“The Climate Grand Challenge I co-lead, Bringing Computation to the Climate Challenge, is attempting to harness AI and other techniques to make climate models faster, easier to run, and more useful to those who historically haven’t been able to do the months-long simulations required,” she says.
“The real promise and impact of AI tools and methods is just beginning to be applied.”
That efficiency boost is likewise enabling physicists to process extensive datasets from experiments and to undertake computationally-intensive theoretical calculations based on the fundamental laws of physics.
Professor of physics Jesse Thaler points out, though, that his field has long employed AI. The seminal discovery of the Higgs boson in 2012, for example, was facilitated by combining several machine learning algorithms to interpret data from the Large Hadron Collider. Physicists rely on AI to make real-time processing decisions about the significant amounts of information particle colliders generate, and Thaler is using it to study the sprays of particles produced by those colliders.
As director, Thaler has framed the NSF Institute for Artificial Intelligence and Fundamental Interactions (IAIFI) around combining the physics tradition of “deep thinking” with the “deep learning” of AI, using neural networks to simulate the brain’s decision making power, to enable “deeper understanding.”
IAIFI researchers are utilizing AI tools to address fundamental physics problems, like the nature of dark matter, and to improve operation of large-scale experiments, like the LIGO gravitational wave observatory. But they’re also applying physics principles, like diffusion and space-time symmetries, to improve AI for applications from materials discovery to video processing.
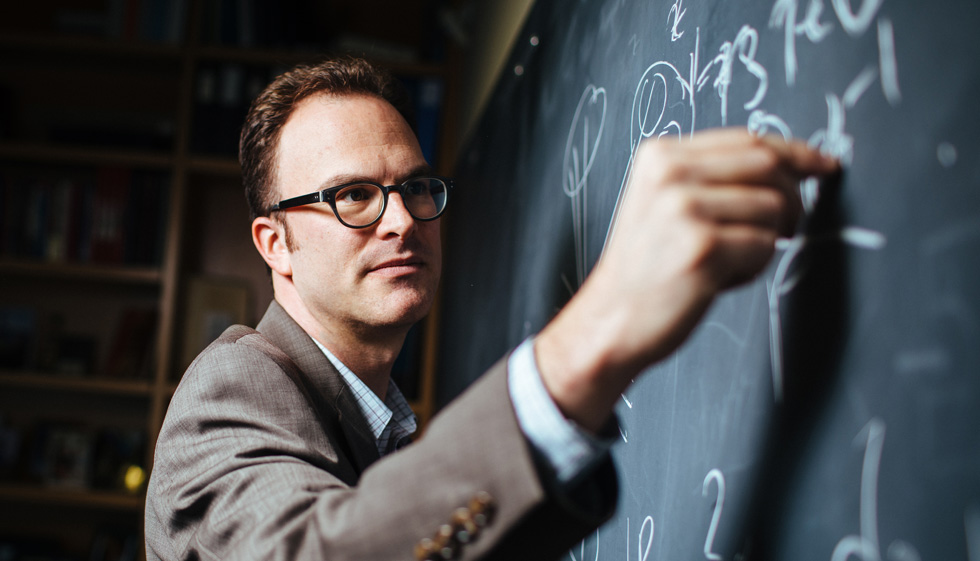
“Instead of treating AI like some inscrutable black box, we can bake physical principles into the AI, to make it ‘think like a physicist,’” he says. “At the same time, I’ve learned more about how to ‘think like a machine’ and leverage the power of computers to solve complex optimization problems whose solutions could not be obtained through traditional techniques.”
That process, he says, often goes both ways, where “AI for physics and physics for AI come together in a virtuous cycle of innovation,” a model that scientists across MIT are replicating.
“By using the language of AI, I often find it easier to talk to scientists in other domains,” Thaler says. “If one can express scientific problems in the abstract language of computation, one can blur the boundaries between disciplines and leverage AI advances to tackle problems across both AI and science.”
Leah Campbell | School of Science