New perspectives on oId questions: AI meets particle physics
A belief in the power of programming to revolutionize particle physics brought Jessie Micallef to IAIFI, a Boston-area research consortium applying cutting-edge technologies to physics data.
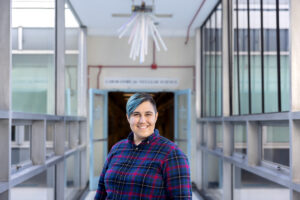
Photo: Steph Stevens
With machine learning, physicists like Jessie Micallef are examining the universe’s most minuscule particles with unprecedented efficiency and power in hopes of unlocking new insights about the forces governing our universe.
“We’re moving from a phase of exploring ways that we can use AI and ways that can help us improve our measurements, to actually regularly doing that,” Micallef says. “That’s going to be a really exciting phase.”
Micallef is a fellow at the National Science Foundation’s AI Institute for Artificial Intelligence and Fundamental Interactions (IAIFI), a collaboration of MIT, Harvard, Northeastern, and Tufts experts applying artificial intelligence to physics research. Through IAIFI, Micallef collaborates with researchers across partner institutions and a tight-knit community of early-career fellows examining everything from astrophysics to particle interactions.
Micallef studies the neutrino, an abundant subatomic particle that remains elusive to scientists.
“While I’m trying to measure a small particle, we have these huge questions that I can help to answer,” Micallef says.
Gaining momentum
Micallef didn’t set out to become a physicist. At their high school in Michigan, they completed three years of both chemistry and physics through the International Baccalaureate program. They entered the University of Michigan to study chemistry.
“Chemistry came a little bit more naturally for me,” Micallef says. Their plans changed when, in their first college physics course, they used programming to model and visualize the effects of forces like gravity. Within weeks, they switched their major to physics.
“Using programming to see invisible physics made it exciting and accessible,” Micallef says. “I decided that studying physics was worth the challenge, exciting, and I was going to put my time into it.”
After declaring their major, Micallef quickly began exploring physics research opportunities. They learned about the field of high-energy physics, where teams of hundreds or even thousands of scientists all collaborate to observe particle interactions. This led them to an internship at Lawrence Berkeley National Lab, which is where they first learned about neutrinos.
Searching for ghost particles
Neutrinos are nearly massless, carry no electrical charge, and rarely interact with other particles, making them difficult to observe and study. They are even invisible to particle detectors. Scientists can only observe neutrinos by spotting the particles created when they interact. Neutrinos are sometimes referred to as “ghost particles.”
“We don’t know a lot of things about neutrinos still,” Micallef says. “And then the more we find out, the more interesting they become.”
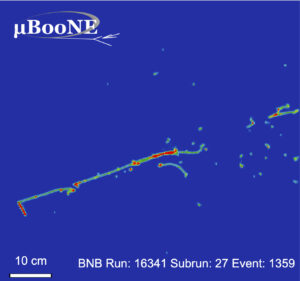
Neutrinos are produced when atomic nuclei interact, which occurs naturally in space at high-energy locations like the Sun. They can also be generated at particle accelerators. While pursuing their PhD at Michigan State University, Micallef primarily worked with data produced at IceCube, a neutrino observatory located at the South Pole which images interactions from neutrinos produced in space. Now, they contribute to MicroBooNE and DUNE, which are accelerator-based detectors at Fermilab in Batavia, Illinois.
“As a prospective mentor, I read Jessie’s application, and was really impressed by what they had done before in the context of IceCube,” says Taritree Wongjirad, an assistant professor of physics and astronomy at Tufts University and Micallef’s IAIFI mentor. “Jessie also had a very clear vision about how to apply machine learning to projects involving accelerator neutrinos to help us achieve our goals.”
Wongjirad, a former MIT Pappalardo Fellow in Physics, is one of a growing number of neutrino physicists integrating machine learning into their work.

“Really precise measurements”
“What we’re trying to figure out by studying the neutrino is why the rules that we’ve established to characterize particle physics do not perfectly describe what we actually end up seeing,” Micallef says.
The universe’s creation should have resulted in equal amounts of matter and antimatter. Yet physicists see stable matter in the observable universe, without any pockets of antimatter.
The Standard Model of particle physics, a set of widely accepted rules that explain most physics observations, is constantly being refined. While the Standard Model predicts neutrinos to be massless, in 2015, the Nobel Prize in physics was awarded to researchers who confirmed they do have mass. Micallef says that knowing neutrinos have mass now opens the possibility for them to have other interesting properties, such as neutrinos and antineutrinos behaving differently. This difference could help explain the matter/anti-matter imbalance in the universe.
Traditionally, to spot and study neutrino interactions, physicists have relied on complex, time-consuming computational programs to examine millions of particles from detector images. Now, researchers including Micallef are building and deploying machine learning tools that sift through these images much more quickly, analyzing each pixel by pixel to categorize interactions and highlight images of interest.
“There are many particles that can come out of a neutrino interaction, such as electrons and protons,” says Micallef. We use these tools to tell us what particles have left a signature in our detector, kind of like using machine learning to tell you if the image has a cat or a dog, but we ask it if it’s an electron or proton.”
Major advances in machine learning have coincided with progress in accelerator and detector technology.
“In neutrino physics, we’re starting to get really precise measurements, which can give us a clear idea of how our observations align with the Standard Model,” Micallef says.
Building connections in science
As Micallef charts a new course at the intersection of machine learning and particle physics, they are also encouraging the next generation to pursue their scientific passions.
“As a collaborator in my group, Jessie was very quick to work with the students and help mentor them,” Wongjirad said. “Jessie is very good at bringing in different people and forming groups to accomplish something that will have a good payoff.”
Throughout graduate school, Micallef regularly volunteered at events and conferences for undergraduate women in physics. They also started a public outreach project called “Portrait of a Scientist.” At conferences and meetings, Micallef asks scientists to fill out and pose for a photo with a sign reading “I am a ______ and I also _______.” Researchers have shared wide-ranging personal interests, from raising chickens to knitting. Micallef assembles these images into collages posted online and on social media to showcase diversity in science. Eventually, they hope to share the collages in classrooms.
“Confidence is such a big thing that can steer people away from any field,” Micallef says. “So many women in physics helped instill confidence in me. I wanted to give back as I’ve gotten later into my career.”
Alison Gold | School of Science